Artificial intelligence and machine learning are often used in the same context. They are related terms but have different purposes. AI has multiple applications across various industries, with the main idea of replicating human behaviors and intelligence in a manufactured way. Machine learning is the method used to train AI behind the scenes, allowing it to learn and carry out tasks independently. Even the most affordable Android smartphones have AI and machine learning elements built in, allowing for unique software-based features beyond the typical hardware limitations.
This guide covers the key differences that AI and machine learning have and gives examples of how they might be used across multiple products or industries.
What is AI, and why do you see it everywhere now?
Artificial intelligence (AI) has recently taken over our world, and no one expected it to dramatically affect our lives the way it has in such a short time. Almost overnight, the term AI is being thrown around everywhere. There isn’t one specific meaning or use case for AI. It’s a generic term. Since AI can be applied to any industry, it has become a universal tool depending on its application and where it’s used. AI is present across various products and industries, including smartphones, healthcare, finance, and marketing.
Source: USAII
Think of AI as a computer model trained using data to help it carry out specific automated tasks. Training an AI model on large data sets is well worth the result since it can accurately and quickly sort through information, surpassing humans. This allows for faster turnarounds that would take an eternity to process using traditional methods. Since a machine can learn and apply what it learned once it reaches the deployment phase, this is part of the appeal of AI. It also doesn’t get tired like a human would.
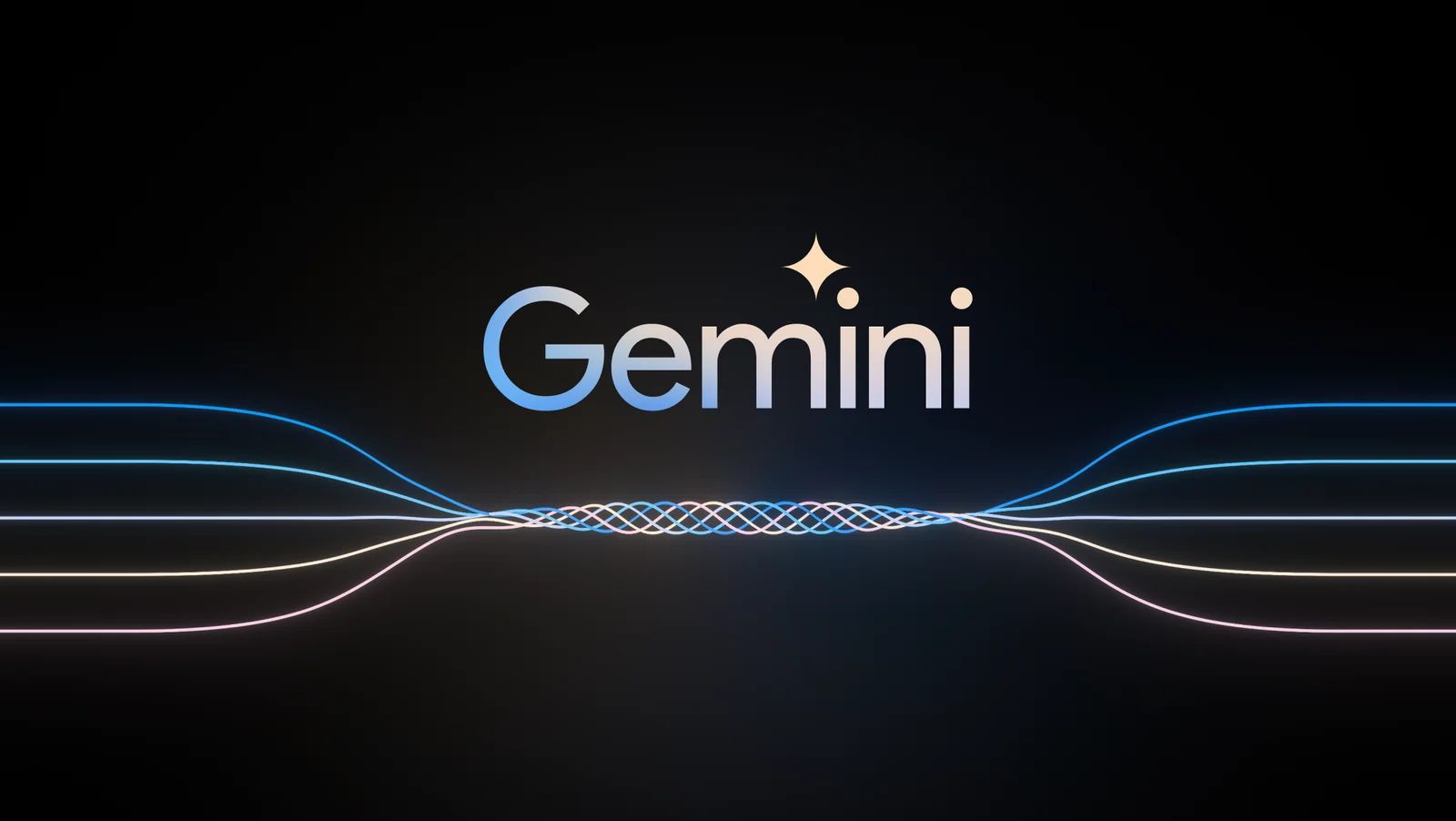
Google Gemini: Everything you need to know about Google’s next-gen multimodal AI
Google Gemini is here, with a whole new approach to multimodal AI: Here’s what you should know.
The initial goal of AI may have been to replicate human intelligence and behaviors as closely as possible in a digital way, but things are starting to change. It now depends on the context or application. AI doesn’t have to be a physical object or a human-sized robot. It could be a digital chatbot, an image upscaler, or an online content moderator. These use AI but have different purposes. No longer just the idea of human-like talking robots. AI has evolved and now encompasses many new aspects of our lives today.
What is machine learning, and how does it differ from AI?
Machine learning is considered a subset of AI, so it is often used in the same context. They are related. Machine learning is one method of training the features of an AI-powered application. It relies on a neural network, which is designed to be like the human brain, with digital nodes representing the neurons. In machine learning, a neural network goes through various stages of learning where these neurons work together in layers to sort information. Using specific predefined algorithms, neural networks learn by repetition, identifying patterns and correlations between the data.
Source: “Machine learning methods for wind turbine condition monitoring: A review” by Stetco et al.
Think of AI as the features you interact with, while machine learning allows many of those features to exist and operate in the background. The more advanced AI features can be powered by the cloud, leaving room for unlimited processing power, as long as your device has an internet connection. At the same time, we’re seeing more on-device machine learning or AI features that rely on dedicated hardware to perform offline tasks and offer unique features. For example, consider the Google Tensor chip in the latest Google Pixel smartphones.
On top of this, Google’s ML Kit allows app developers to squeeze lightweight but powerful on-device machine learning models into their apps. Any app developer can add machine learning features while relying only on the processing power of your device. It’s entirely offline. Some examples of machine learning features ML Kit offers include image recognition, text translations, and identifying products on a store shelf. Since they don’t need a constant internet connection, the response times are faster, and they don’t use mobile data.
What are some applications of AI today?
Since AI covers various applications and industries, we’ll give a few examples of where AI is used today. Many other examples exist, but we want to point out a handful below.
Chatbots and their impact on our devices
AI-powered chatbots are some of the significant applications that have taken over the consumer market in record time. The biggest names include ChatGPT, DALL-E, Google Bard, and Microsoft Copilot. These chatbots use generative AI, which is the next evolution of chatbot technology. You can ask it to do something, such as answer a question or create a wallpaper for your smartphone, and it generates it for you in real time. You can research topics without spending hours sorting through websites or be creative without being a professional artist.
Smartphone cameras take better photos
The Google Pixel smartphones have always had AI-powered cameras and machine learning features since the original device in 2016. Their unique HDR+ image processing technique allows the camera to take even better photos than the hardware is capable of. It takes multiple images with different exposures and stitches them together using AI to create a cleaner, higher-quality photo. This is why they outpaced some dual-lens smartphones using a single lens. Google also continues adding new AI camera features to its latest Pixel phones, such as Photo Unblur, Best Take, and Magic Audio Eraser.
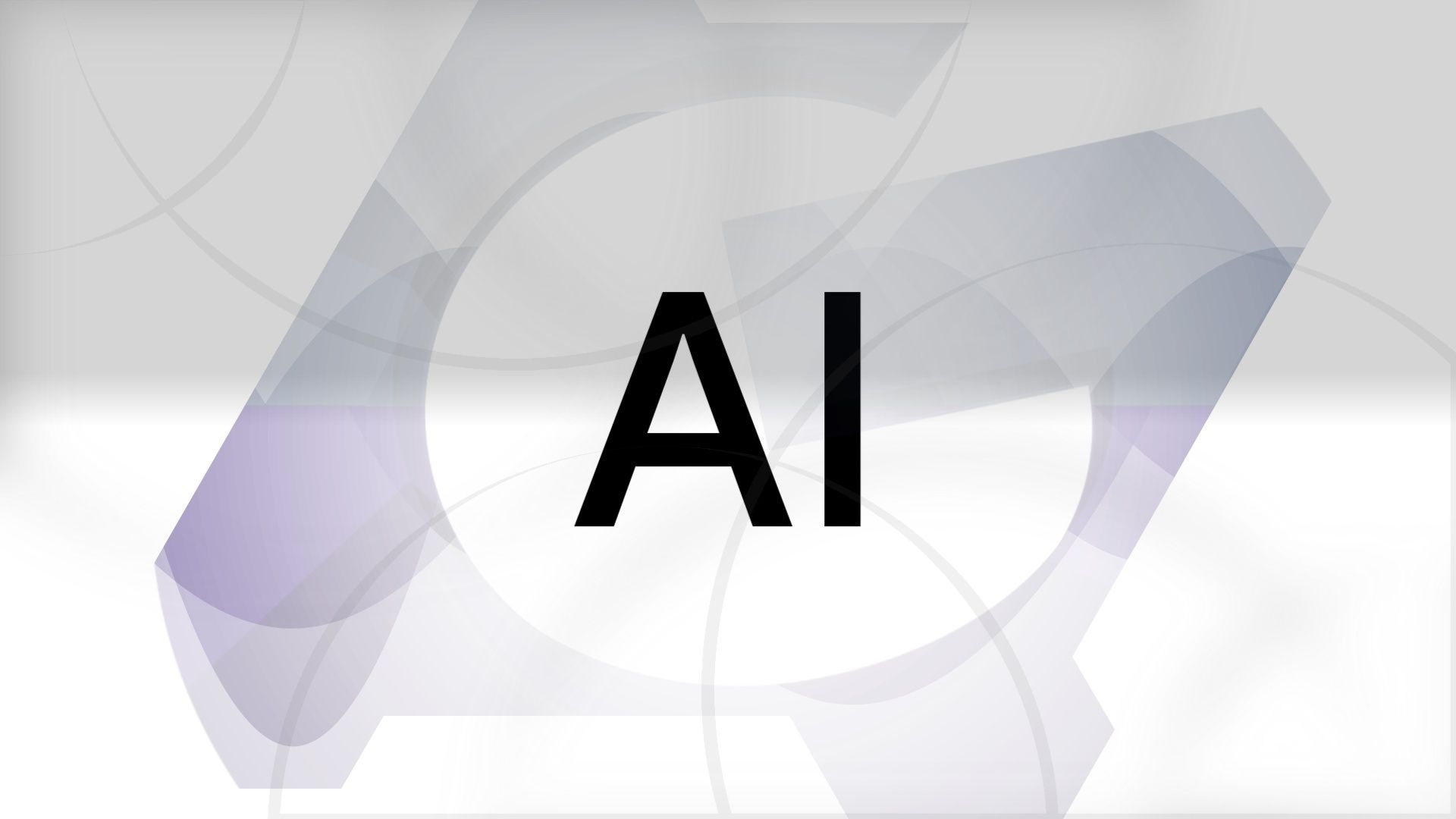
What is a vision model?
Powering many of the image-based and AI applications today
Apple, Samsung, and other big smartphone manufacturers also rely on AI and machine learning features in their cameras. When paired with new larger camera sensors, AI’s power and efficiency help us take better photos and videos. For photos, this is known as computational photography. Although imperfect, the vision models and algorithms that power these AI and machine learning camera features continue to improve.
Social media platforms and content management
With social media being such a large industry, there needs to be a way to moderate content responsibly. Facebook, Instagram, and WhatsApp use AI systems to flag content that may be offensive or inappropriate. Their AI scans for offending posts at all hours of the day and night, more than humanly possible. This method isn’t always perfect. The AI can sometimes flag content that it shouldn’t. Various human reviewers often come in after the AI to ensure everything checks out after a post is flagged.
Banking and credit cards offer enhanced security
Source: Affirm
Many of us have online banking or credit cards, which we use when buying items online. For extra security, set up two-factor authentication (2FA) or passkeys on your accounts, lock your financial apps with your fingerprint, and create a virtual card. But what about the things you can’t control? Some banks and credit card companies are deploying AI and machine learning systems to detect and track fraudulent customer charges. Their systems catch and flag fraudulent charges in real time using various computer models, statistics about recent purchases, and algorithms.
Gaming now supports AI upscaling features
Source: Google
Experiencing a realistic gaming world with smooth performance requires significant processing power, which can be challenging, even for a gaming PC. Things become more complicated when you increase the resolution, add highly detailed textures, and toss in demanding ray tracing. Upscaling technologies like DLSS, FSR, and XeSS achieve that performance goal with minimal loss in visual quality.
AI-powered upscaling for gaming is fascinating since it relies on deep learning, a subset of machine learning. Deep learning is beneficial for language translation, object detection, and image recognition, which are perfect for video games. On top of that, DLSS, for example, uses specialized AI cores on NVIDIA GeForce RTX graphics cards, offloading some workload from the GPU and freeing up system resources. This allows games to offer higher performance without stressing the GPU as much, resulting in greater efficiency even on lower-end machines.
It won’t be long before we see gaming consoles and smartphones using built-in AI chips dedicated to upscaling games. AI-powered upscaling features are said to play a vital role in the upcoming gaming consoles in 2024 and beyond. This can help games push higher performance goals, maintain a stable frame rate with high graphic fidelity, and include more ray tracing features. A giant leap forward for the gaming industry. Combining raw hardware power with AI can create efficiency beyond the typical hardware limitations. This will benefit all types of games, including flat-screen, VR, and mobile.
Of the three upscaling technologies for gaming, only DLSS and XeSS use or offer AI-powered features. FSR relies on software-based upscaling methods, which gives it the most compatibility across hardware, including current-generation consoles. This method works well without relying on AI, but FSR may introduce AI features later.
Healthcare is more accurate and personal
The healthcare industry benefits when paired with AI, which makes it another perfect use-case scenario. For example, using an AI-powered algorithm to identify irregularities in an X-ray or CT scan is faster and more efficient than its human counterparts. Since the AI is trained on a comprehensive data set beforehand, it knows exactly what to look for with immense speed and accuracy. At the same time, doctors can use AI to scan a patient’s data into their system and have it generate a personalized treatment plan.
Autonomous and predictive data marketing features
Marketing is a tricky business to get into since you must understand trends and learn what works and what doesn’t. It’s constantly changing and evolving. The marketing industry and AI work well together for data analysis. AI can analyze customer data and create a campaign designed for that group. AI can also give accurate ad bids for popular advertising platforms to get the most from an ad campaign budget. It even predicts future customer behaviors using previous data and patterns from the analysis.
AI and machine learning are here to stay
AI is the general term that gives unique features to devices, applications, or products after they are trained to carry out specific tasks. Machine learning is a method that trains and powers these AI features. The AI and machine learning boom is only getting started. With companies, products, and industries gearing up to invest heavily in AI technologies, AI will expand to other parts of our lives. We’ll soon see more products with dedicated AI-powered chips and features, from smartphones and gaming consoles to smart home devices.
Machine learning models can power AI, but large language models (LLMs) are the backbone of AI-based chatbots. We explain everything you need to know about LLMs and why chatbots like ChatGPT or Google Bard rely on them.